3 Reasons Why Retailers Are Stocking Up on Machine Learning
The aura of anticipation and uncertainty that permeates discussions around AI and machine learning tends to overshadow the near – term and practical use cases of the technology. One space already showing the promise of machine learning is retail. Today more than ever, the customer is king as shoppers expect on-demand, seamless and personalized buying experiences. Machine learning can aid in getting retailers to meet these expectations, but first it’s important for decision-makers to look beyond ‘AI washing’ and understand the current opportunities of where machine learning can be applied to retail use cases.
The fact that the digital and physical worlds are colliding is felt most acutely in retail. It’s virtually impossible to plan for all potential customer journeys, because each is essentially a non-linear, self-directed interaction, or ‘micro moment,’ across a customer’s channel of choice. Furthermore, the growth in customer data coupled with the maturation of machine learning and AI ensures that retail is well-placed to benefit from new disruptive technologies that boost efficiency and improve customer experience.
Our Voice of the Connected User Landscape’s 2H 2017 Digital Transformation Survey illustrates a huge potential for how retailers are most interested in exploring advancements in Machine learning and AI as potential retail game-changers over the next 24 months. Our survey states that retailers are highly interested in use cases, which prioritize operational efficiency and customer engagement.
Figure 1: Artificial Intelligence and Machine Learning Opportunities in Retail
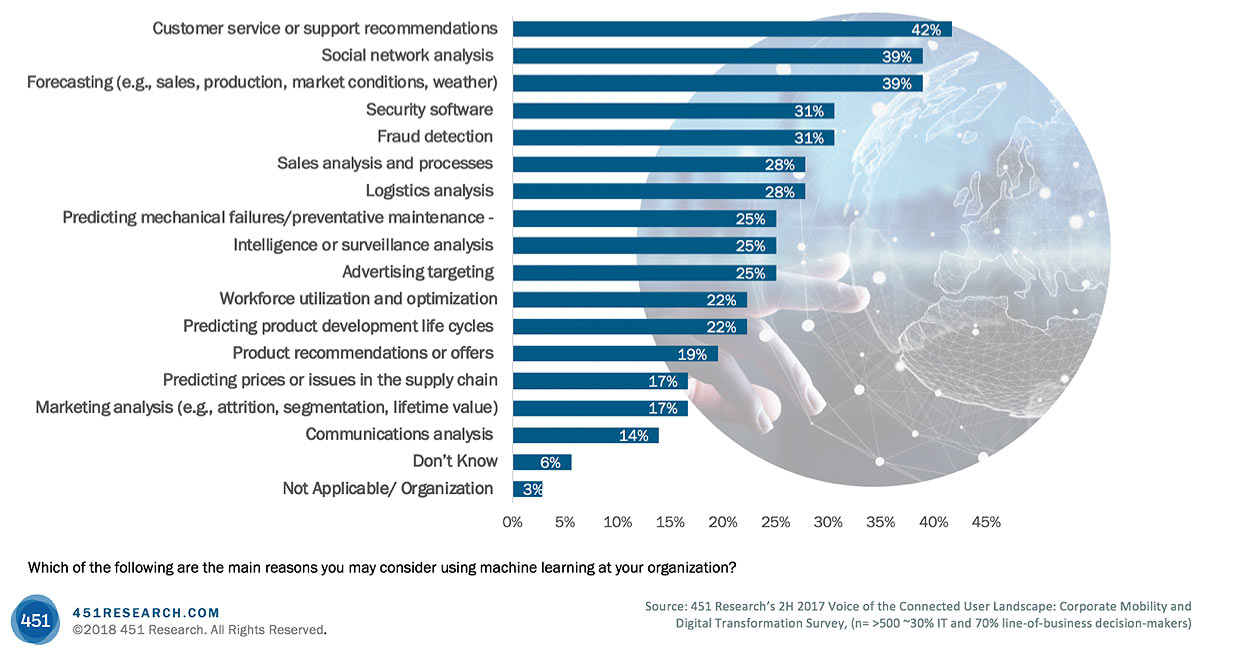
Retailers are looking to move beyond segment-based rules analysis toward algorithmic decision-making that enable hyper-personalization at scale that factor in individual affinity along with overall intent, resulting in greater relevancy and effectiveness. Self-learning algorithms allow marketers to auto-adjust adjust or adapt based on any one or any combination of factors such as individual customer or visitors’ behavior, geolocation, inventory levels and manufacturer incentives etc.
So, what can retailers do with machine learning for improving customer experience?
Increase Customer Engagement
The customer-engagement segment involves the use of machine-learning-supported technologies that improve the overall buyer experience, whether online, mobile or in-store. Using AI and machine can create a truly unique immersive experience for customers. There are also ways to improve customer service through improved self-service capabilities through a conversational experience that provides automated, personalized selling or VIP support experiences. Customers can get help with products, track shipping status and product returns all within the confines of an automated digital conversation. If escalated to a customer service representative, recommended responses help expedite resolutions to improve engagement and lower operational costs.
Optimize In-store Operations
There is a wealth of opportunity to improve in- store operations. Efficiency of the operating model is everything in retail. The ability to pick up on small drivers of change at the very earliest stage, and then finesse operations in response to those changes is what makes for a well-oiled retail machine. We know that a small modification such as a placement of a product can provide competitive advantage. The slightest rerouting of a pick list to speed delivery can reduce shipping costs. The improvement payment processes, streamlining checkout experiences and overall improvement of in-store efficiency is categorized under the in-store operations segment.
Reduce Fraud
The retail industry depends on secure transactions and transparency. Currently, one of the primary uses of ML is reducing manual reviews of suspicious transactions (e.g. billing/shipping address mismatch, cross-border purchases) by taking humans out of the process and making better informed decisions based on previous transactions across numerous different merchants. Behavioral biometrics is another use case for machine learning, which compares a users’ behavior to previous interactions with other shoppers (or bots) that have results in fraud. Counterfeit identification and product authentication is another area relying on machine learning to accurately detect bad-faith commerce.
As technology becomes increasingly central to how individuals experience the world around them, it is shifting the center of gravity from businesses to individuals in an unprecedented way. New AI and Machine learning technologies can help businesses meet rising customer expectations as they expect an intelligent, immersive experiences, all personalized to their own changing context.