Blasting Through the Four Biggest Challenges to Leveraging AI in Financial Services
Artificial intelligence (AI) is taking all industries by storm – including financial services – but it’s a cautionary tale. Those looking to deploy AI and Machine Learning (ML) must avoid buying into the hype and understand it actually takes a lot of work to build a successful AI system.
In a recent webinar on AI in the financial services industry, Stuart Tarmy, Aerospike’s global director of financial services industry solutions, explained that AI is currently used in a wide variety of financial service areas, from full-service and online brokerage firms to insurance to banks. In addition, a McKinsey study finds that most high-performing companies have boosted their investment in AI during COVID-19, with financial services increasing by 28 percent.
“The takeaway message here is that forward-thinking companies are rapidly adopting AI and you really need to learn to embrace it, or you’ll be left behind,” Tarmy says. “What we’re seeing in the research is that companies are becoming bifurcated. The winners are winning more, and the people who are not keeping up are falling further behind in their growth and performance.”
Still, Tarmy pointed out in his presentation that there are multiple fronts that must be addressed if companies want to use AI to their advantage.
1. Using advances in AI to significantly improve model development and performance.
Tarmy says “best of breed” AI systems need great data scientists who can build top-notch AI algorithms and the ability to use large volumes of data (terabytes, petabytes and exabytes), “because the more data, the better you can tune the system,” he says.
It is essential to have a super fast engine so that you can do real-time processing.
“In many of these cases, you want to be able to provide real-time experience to the business units or to the customers, so we’re talking milliseconds in terms of performance,” he says.
Aerospike can serve as the intraday system of record, handling millions of transactions per day that are executed from mobile devices, PCs, ATMs and branch offices. At the same time, it can be a risk modeling and analysis tool for financial institutions that need an integrated, real-time view of the market, credit and liquidity risk exposure across asset classes and customers. It can also provide identity management, fraud detection, personalization and compliance features.
Further, Aerospike can step in and help firms with applications needed by various teams, whether they’re in the front, middle or back office. From portfolio optimization to compliance to workflow accounting, Aerospike can provide real-time, reliable, fast performance over extremely large data sets, Tarmy says.
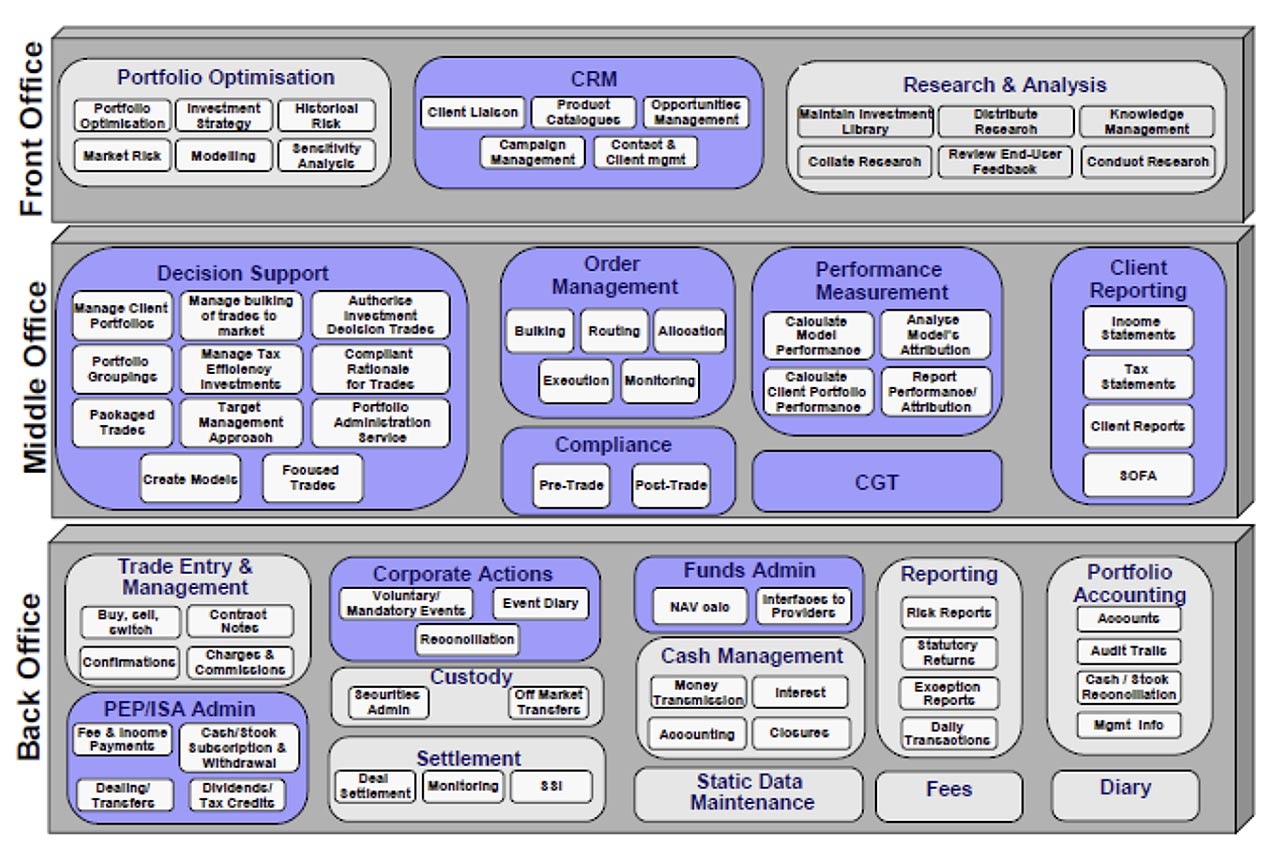
Aerospike can meet various challenges posed by different teams
2. Bringing in large, multiple types of data is essential to improving AI-based system performance.
The most advanced companies have moved to neural nets and deep learning systems. Neural nets work on a concept of neurons talking to each other – similar to the human brain – only this is inside a computer system. Companies using them are analyzing a million to 10 million attributes.
For example, PayPal has moved to neural nets, and report they have improved performance by 30 percent compared to when they were using an AI/ML-based system.
In addition, more companies are exploring explainable AI, which builds an AI system on top of an expert system to provide explanations of why things are being done, Tarmy says.
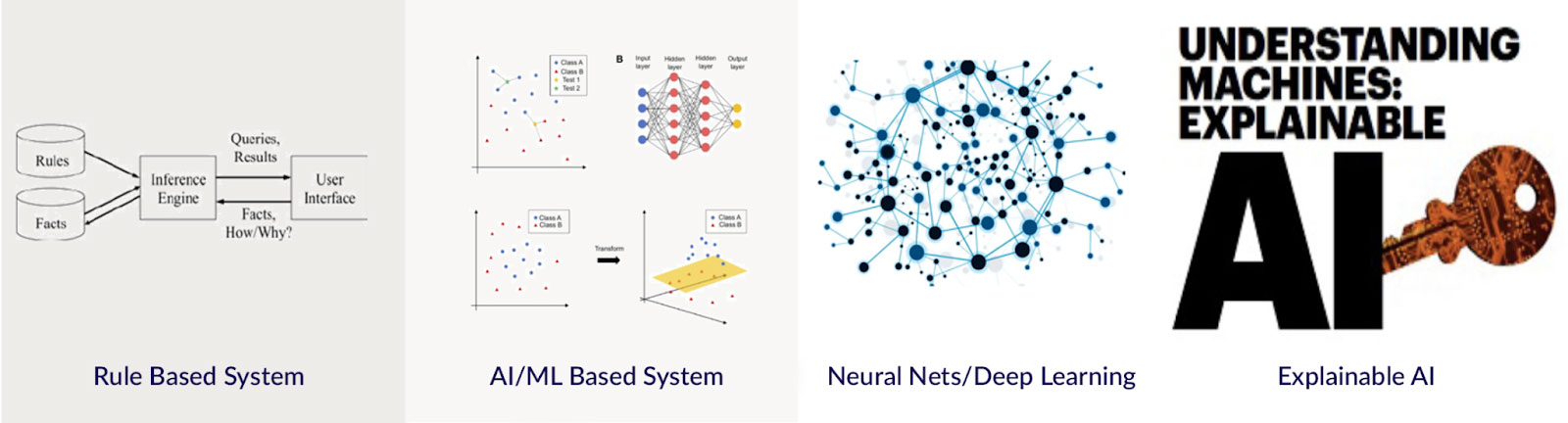
Explainable AI is important because it allows companies to build better systems. “The more you understand what’s going on inside the black box, the better you can tweak the system, and tune it and understand what attributes are working. You may find ones that you don’t want to include or that you want to include,” Tarmy says.
Another key ingredient of explainable AI is that it enables companies to provide faster, reasonable explanations to users of how their data will be used, which is now required under various data privacy rules in California and in Europe.
3. How to build and deploy leading edge AI-based applications for real-time performance.
Aerospike’s architecture can not only support fast model testing, but can ingest data from disparate data sources at the edge for systems of engagement in real-time, which is critical in providing better customer experiences.
Aerospike can be used for business platforms in areas such as consumer lending and credit cards; in enterprise platforms that are used throughout an organization for analytics and data or payments; and enabling platforms that are used for cybersecurity and general IT infrastructure.
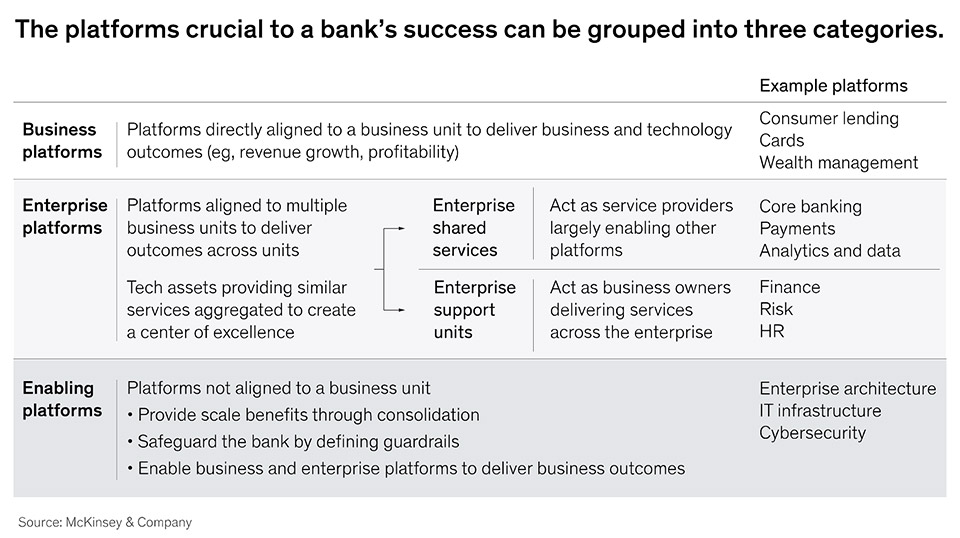
“The total cost of ownership and the amount of servers you need to do this is greatly reduced from other solutions,” Tarmy says of Aerospike.
4. Why use AI in a multi-cloud, on premises hybrid environment.
A multi-cloud, on premises hybrid environment provides flexibility for those financial institutions that may still have a legacy system, don’t want to get locked into just one cloud vendor, want more negotiating room on cost or would prefer – for data privacy reasons – to have an on-premises solution.
“The good thing about Aerospike is that it is a software layer. We enable companies to run across all of these scenarios – multi-cloud, on-prem, hybrid – and make it relatively straightforward,” Tarmy says.
At the same time, Aerospike is “active-active,” which means that it’s always on and staying consistent throughout the database, along with multi-site clustering that allows you to put clusters in different data centers and keep them consistent.
“We can also work across data centers and have everything communicate in a very smooth and consistent way,” he says.
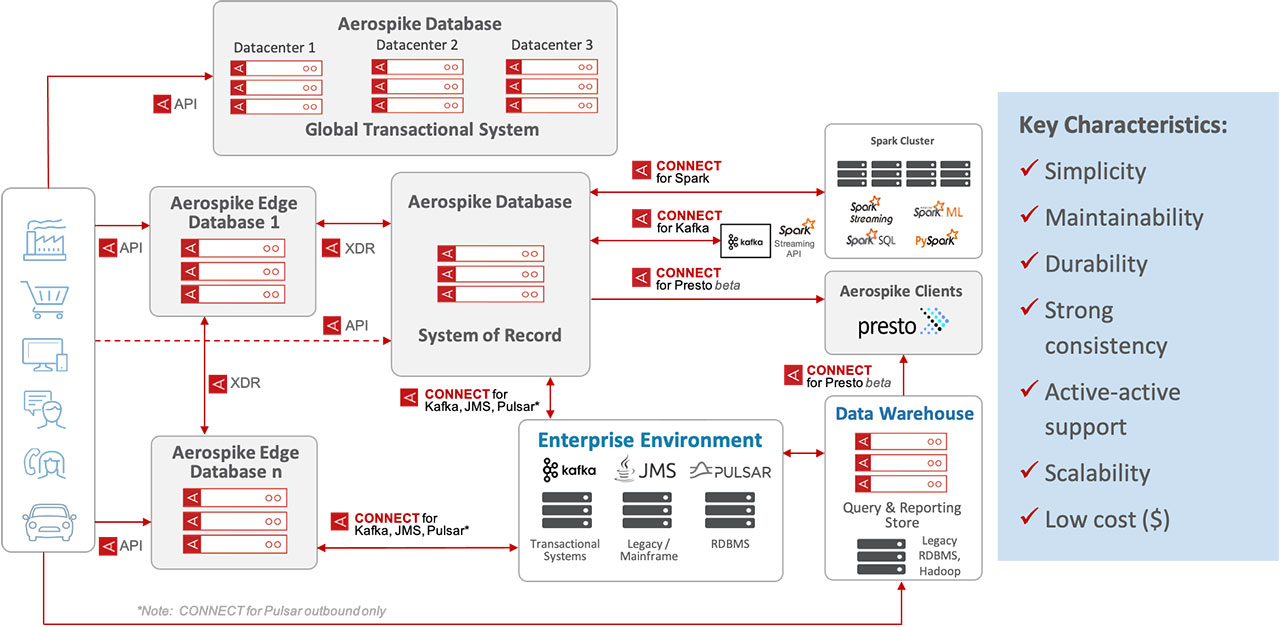
Aerospike Real-time Data Platform
For companies like Signal, which is a SaaS provider of data onboarding and real-time identity resolution, Aerospike’s total cost of ownership and reduction of servers from 450 to 60 saved the company about $6.9 million over three years, Tarmy says.
In addition, Signal’s throughput went from 500,000 transactions per second to eight million, and latency dropped from 3,900 milliseconds to 23 milliseconds.
Finally, as Signal and other companies such as PayPal show, Aerospike’s lower TCO, high throughput, low latency, reliability, and consistency can provide financial services companies with efficient and fast solutions that help them solve their greatest AI challenges.
Watch the full webinar replay of Stuart discussing these issues as well as many others.