Real-time personalized homepages for improved customer experiences
About Myntra
Myntra began in 2007 as a shop that personalized gifts and grew into a major Indian fashion e-commerce company focused on making the world a more stylish, colorful, and happier place. With $425M in revenue and 11,000 employees, they use technology to help people look great as well as democratize fashion and lifestyle. Myntra provides best in class customer experiences with one of the richest fashion product lineups in the country, including in-house and exclusive brands such as Roadster, HRX, and House of Pataudi.
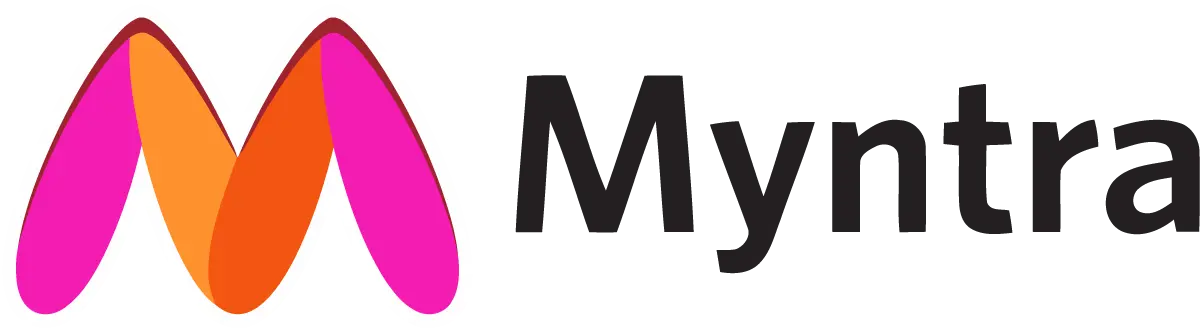
Bottlenecked feature store lookup process was hurting the customer experience
Myntra develops a deep understanding of their customer to provide a real time, personalized shopping experience that recommends sizing, styles, brands, and products specifically tailored to individual preferences. When a customer opens the Myntra homepage, Myntra uses a machine learning (ML) ranking model created from its feature store to populate the screen with widgets aimed at improving the customer experience, resulting in high click-through rates and revenue.
Myntra’s model relied on feature lookup processes built on Redis to accurately build the experience. With hundreds of millions of users, 20K orders per minute at peak, a million active users per day, and over 500K concurrent users during peak events like sales, the feature lookup process was taking too long to execute, resulting in poor user experience. Myntra needed a different approach to accommodate the scale and performance they required to serve their growing customer base. Their needs included:
Speed and scale
Deliver homepage personalizations at throughputs of 100K-400K requests per minute and latencies under 40ms at the 99th percentile.
Eliminate bottlenecks in the feature store
Fetch features and embeddings in real time with low latency.
Consolidate the tech stack
Simplify the Azure technology stack that included petabytes of data stored across multiple SQL, NoSQL, and cache systems powering ~500 services, data science, machine learning, and observability.
Improved user experience, CTR, and revenue
After discovering through evaluation that Aerospike’s enterprise solution would help them overcome Myntra’s limitations in namespace, node expansions, scaling, and infrastructure costs, Myntra migrated more than 25 clusters and hundreds of services onto the Aerospike Database Enterprise Edition. The migration was seamless with zero downtime and kept everything in sync.
As a result, Aerospike significantly improved the performance and scalability of their feature lookup, resulting in improved customer experience, higher click-through rates (CTR), and stronger revenue. The solution with Aerospike delivered:
P99 response times under 5ms
Aerospike excelled in performance compared to other data stores and provides P99 response times of less than 5ms
Efficient, consolidated footprint
Reduced to 8TB, 25 production clusters, 150 different nodes and overall reduction in cloud resource footprint
Cross datacenter replication (XDR) and strong consistency
Hybrid Memory Architecture™ (HMA) allowed data to be kept on SSD and primary index in memory
Strong partnership
Exceptional support and engineering involvement with quick response and resolution times; willingness to help with new use cases
Reliable performance in business-critical services
Today, Myntra views Aerospike as a trusted partner with reliable database technology for their most critical workloads. Myntra plans to expand their use of Aerospike, continuing to consolidate clusters, expand their use of XDR to improve disaster recovery preparedness, leverage enterprise security and encryption capabilities, and potentially upgrade to a container based architecture. Current uses include:
Millions of search operations per second
Search is a business-critical service that requires millions of operations per second and P99 of under 1ms reads
500K personalization operations per second
Supports half a million operations per second at sub millisecond response times for homepage personalization
Billions of notifications daily
Sends out a billion notifications every day with users receiving, on average, 5 notifications a day, all processed within a 2-3 minute window
Millions of transactions per second
Builds ML models from features and is spread over 8 clusters with tens of millions of transactions per second
Testimonials
Additional resources
For a deeper understanding and more insights, explore these additional resources.
Get started with Aerospike
For high-performance, scalable data management and ultra-low latency, ideal for handling massive datasets and real-time applications.